Strategic Data Management for AI
A Comprehensive Guide to Building Data Infrastructure for Enterprise AI
Abstract
This comprehensive guide addresses the fundamental data management challenges that organizations face when implementing AI solutions. It provides a strategic framework for building a robust data infrastructure that supports AI initiatives at scale, covering data governance, architecture, quality, and security considerations essential for successful AI deployments.
Key Components
Business-Aligned Data Strategy
Data Governance Framework
Modern Data Architecture
Data Quality Management
Data Security & Compliance
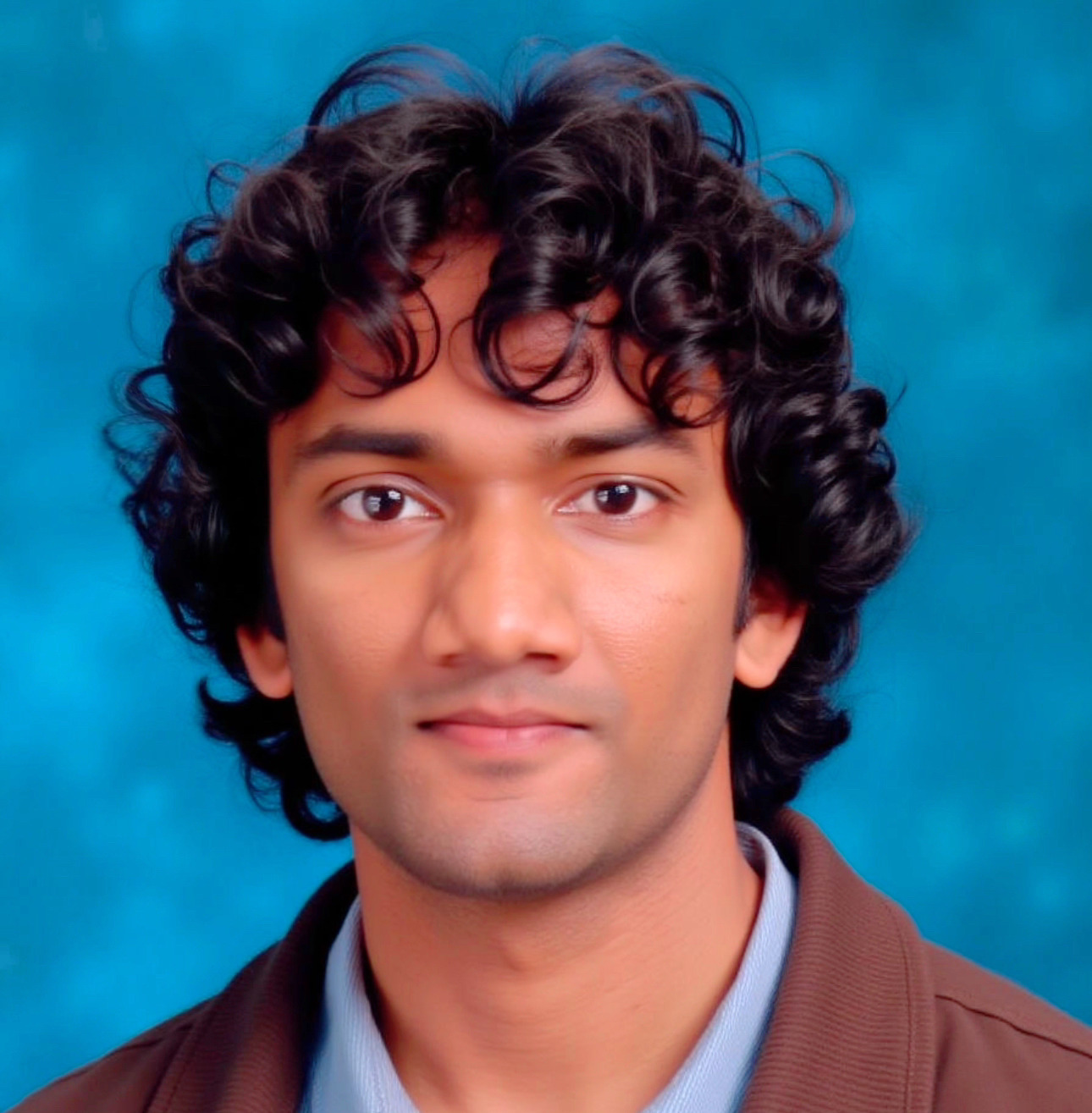
Nim Hewage
Co-founder & AI Strategy Consultant
Over 13 years of experience implementing AI solutions across Global Fortune 500 companies and startups. Specializes in enterprise-scale AI transformation, MLOps architecture, and AI governance frameworks.
Publication Date: March 2025
← Back to Learning HubIntroduction: The Data Foundation for AI Success
Artificial Intelligence (AI) promises transformative business outcomes, but many organizations discover a harsh reality: AI initiatives are only as good as the data that powers them. According to Gartner, nearly 85% of AI projects fail, with poor data quality and inadequate data infrastructure cited as primary reasons.
This guide addresses the critical data challenges that organizations must solve to successfully implement and scale AI. While much attention focuses on model development and deployment, the underlying data foundation often receives insufficient strategic consideration.
AI requires not just more data, but better data—properly governed, accessible, high-quality, and secure. Organizations that address data strategy as a prerequisite to AI implementation experience 3x higher success rates and significantly faster time-to-value for their AI investments.
The sections that follow provide a comprehensive framework for building a robust data foundation that can support enterprise AI at scale, covering governance, architecture, quality management, and security considerations essential for AI success.
References
- [1]
Gartner, Inc. (2023). Top Strategic Technology Trends for 2024: AI Trust, Risk and Security Management. Gartner Research. Retrieved from https://www.gartner.com/en/documents/4258571
- [2]
Bean, R. (2021). Why AI Initiatives Fail. Harvard Business Review, 45-52. Retrieved from https://hbr.org/2021/07/why-ai-initiatives-fail
- [3]
Davenport, T. H., & Bean, R. (2022). Data Governance in the Age of AI. MIT Sloan Management Review, 63(3), 8-11.
- [4]
Deloitte Insights (2023). State of AI in the Enterprise, 7th Edition. Deloitte Consulting LLP. Retrieved from https://www2.deloitte.com/us/en/insights/focus/ai-adoption-in-the-enterprise.html
- [5]
McKinsey Global Institute (2023). The Economic Potential of Generative AI: The Next Productivity Frontier. McKinsey & Company. Retrieved from https://www.mckinsey.com/capabilities/mckinsey-digital/our-insights/the-economic-potential-of-generative-ai-the-next-productivity-frontier
- [6]
Dehghani, Z. (2022). Data Mesh: Delivering Data-Driven Value at Scale. O'Reilly Media.
- [7]
Stanford HAI (2023). Artificial Intelligence Index Report 2023. Stanford University Human-Centered Artificial Intelligence. Retrieved from https://aiindex.stanford.edu/report/
Related Resources
Need Expert Guidance on Your Data Strategy?
Our team of data strategy consultants can help you build a solid data foundation for your AI initiatives with customized guidance and implementation support.